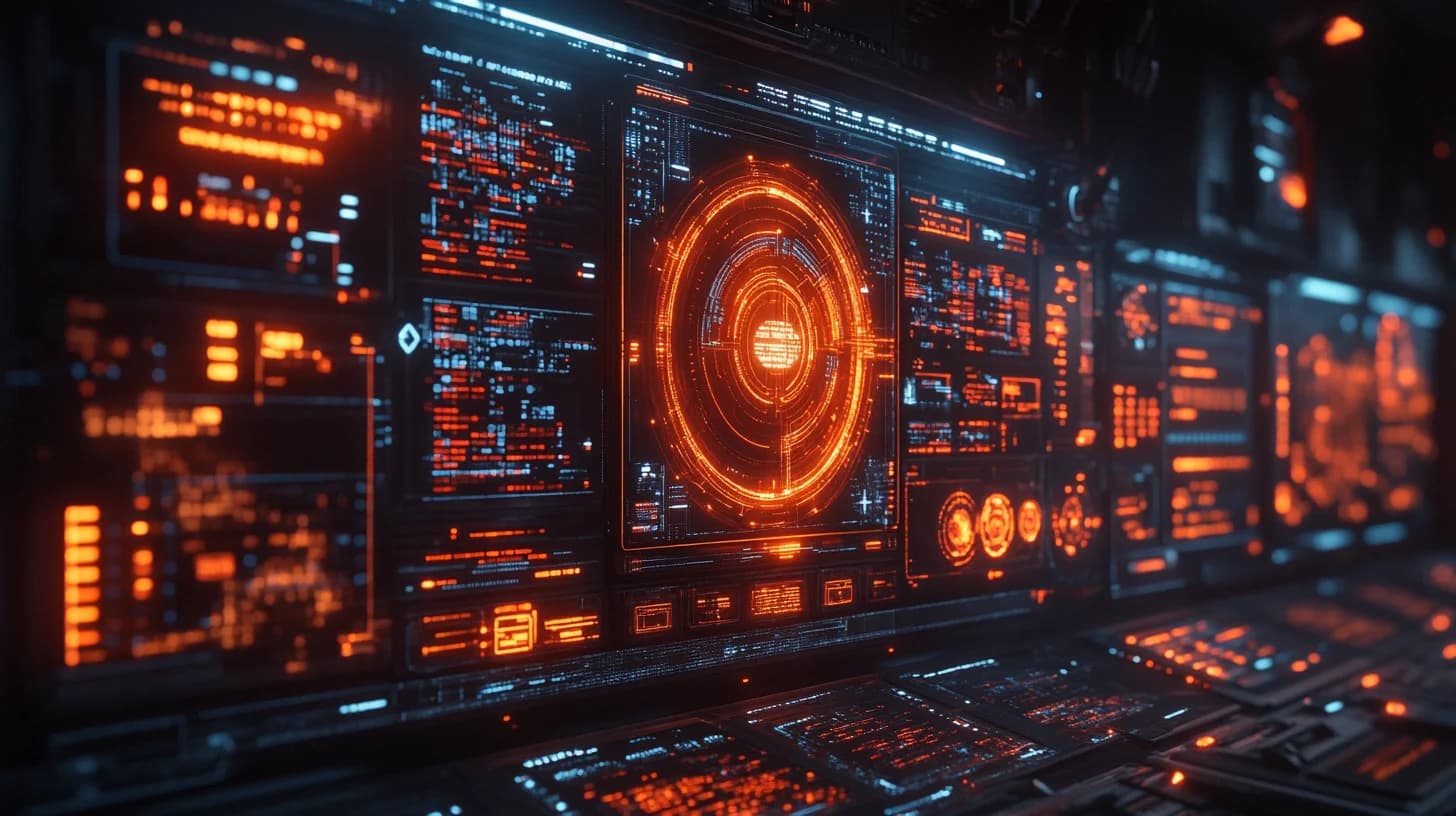
Do Recommendation Systems Work: A Field Experiment
Published on Nov 22, 2022
Abstract
Recommendation engines are ubiquitous on digital platforms. A large section of work has happened on designing them in terms of building predictive models. However, to the best of our knowledge, we do not find studies that compare two recommendation engines on customers using a field experiment. In this paper, we work with our collaborative partner Careem, the largest ridesharing firm in the middle east, to obtain data for a field experiment that tests the differences between two types of recommendation engines – a) one focuses on exploration that shows random widget and b) the other focuses on exploitation and shows intent-based widget on the smartphone application of its customers. Our results show that both recommendations can provide significant impacts (in terms of engagement on the platform through increased app usage as well as usage on the platform through the spending on the platform services) for users that have used widget in the past. However, intent-based recommendation engine performs better than an engine that provides random recommendations. Sub-sample analysis reveals that in some cases, a random recommendation is better than a static recommendation, which is quite counterintuitive and quite beneficial for small firms who can not afford a large data science team to develop and maintain a complex recommendation engine.
Keywords: Nudges, Recommendation, Behavioral Operations Management, Field Experiments